About Me
My name is Dr. Meagan Carney and I work as a Senior Lecturer in STEM at the University of Queensland .
As a mathematician, I focus on the statistical analysis of extreme events in systems like climate and virology. My research includes modeling extreme weather phenomena, applying machine learning to SARS-CoV-2 data, and delving into the statistical properties of extreme events across various systems. I extend extreme value theory to physical observables, aiming to inform our understanding and response to rare events.
Working in Close collaboration with Short lab!
Eveniet quasi nobis aliquid cumque officiis sed rem iure ipsa! Praesentium ratione atque dolorem
Lorem ipsum dolor sit amet, consectetur adipisicing elit. Fugit dolorum itaque qui unde quisquam consequatur autem. Eveniet quasi nobis aliquid cumque officiis sed rem iure ipsa! Praesentium ratione atque dolorem?
Upcoming Events
International Women in Mathematics Day
The University of Queensland (UQ), Queensland University of Technology (QUT), and Griffith University with financial support from Women in Mathematics Special Interest Group (WIMSIG) are co-hosting a half-day International Women in Mathematics Day (IWiMD) event on 31st March 2025 at the Ship Inn, South Bank. While each co-organising university has held IWiMD events in the past, this will be the first Brisbane multi-university event for IWiMD.
Learn more and Celebrate!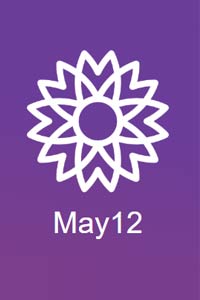